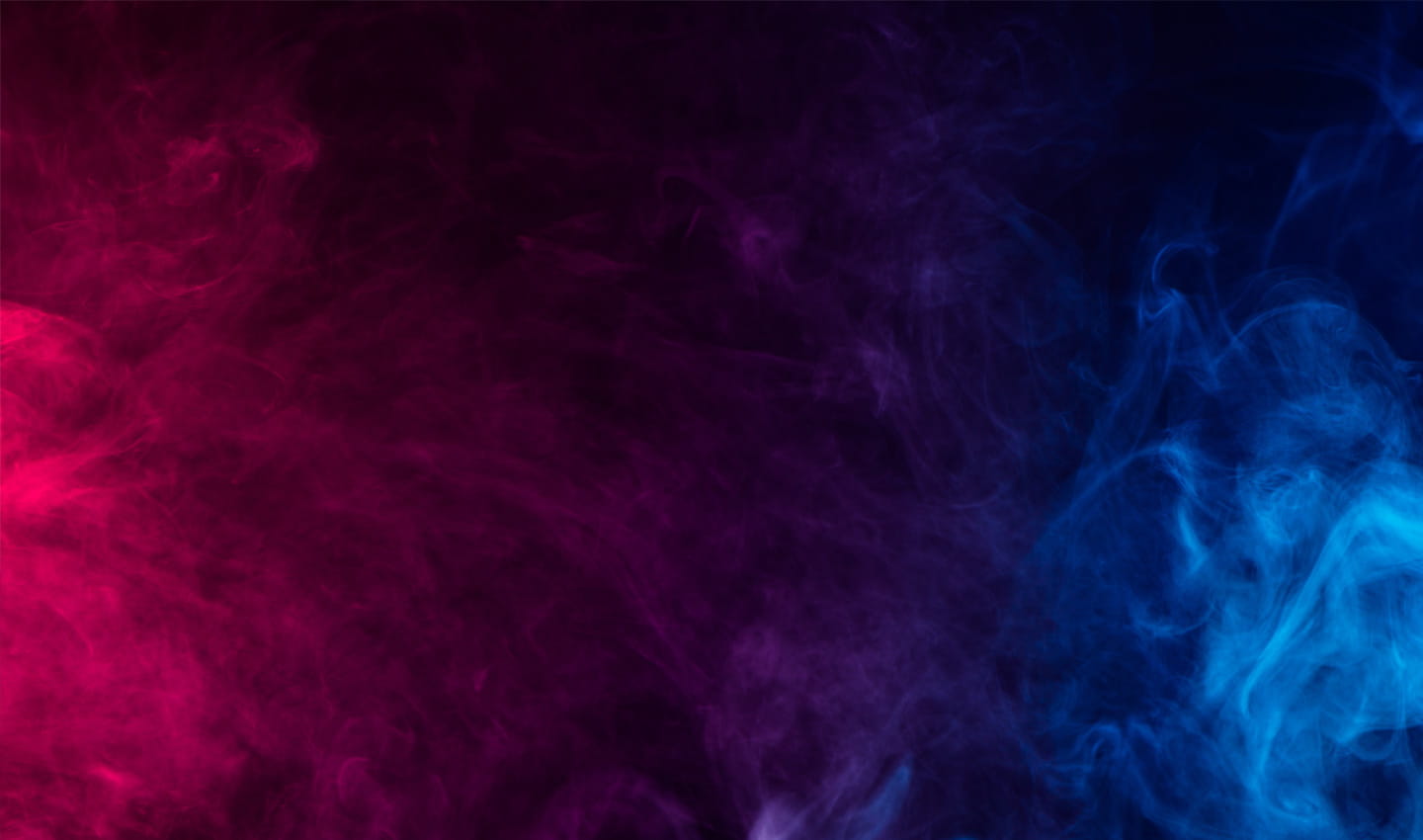
The domain name is parked using the Epik parking platform.
For more information about this domain
The domain you are buying is delivered upon purchase.
Buy with confidence. Your purchase is secured by Epik.
Free WHOIS privacy, free forwarding, 24/7 Support are all standard.